Biased Artificial Intelligence
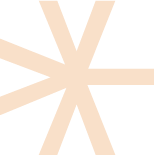
Machine Learning - currently debated under the term Artificial Intelligence (AI) - is entering a new era. This photo, for example, was generated by an AI image generator. But what pitfalls might there be on a societal level?
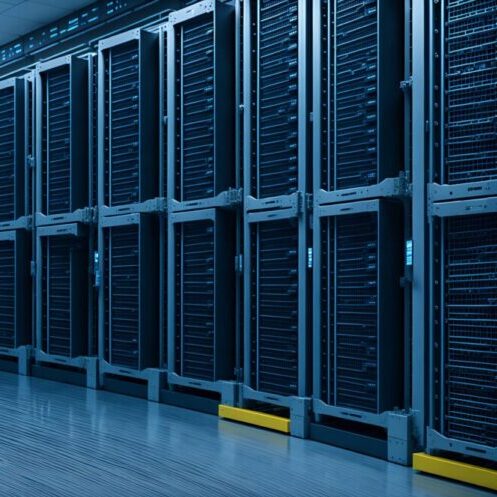
Asked whether it is biased, ChatGPT, an AI (Artificial Intelligence) tool, responded on April 8, 2024: “Like many AI models, ChatGPT can be susceptible to biases. Bias can arise in various ways, such as how the training data was collected, or the inherent biases present in the texts used to train the model.”
Bias in AI tools can be explained by the data used to develop these machines. This data is often not representative and is based on the opinions and attitudes of majority groups. For example, in 2022, the European Institute for Gender Equality (EIGE) reported a share of 18.9% of female IT specialists across Europe. Consequently, stereotypical views towards certain genders, ethnicities, or social groups are often present in the training data, leading AI models to reproduce and reinforce these biases in their widespread applications.
The data used to train tools like ChatGPT primarily come from internet sources such as Wikipedia, an encyclopedia that has historically shown a significantly lower participation of women compared to men since its inception. In 2018, there was a notable gender imbalance in terms of who contributes to Wikipedia, with the Community Insights Report indicating 90% male contributors, 9% female contributors, and 1% other individuals.
While this is not new information, avoiding these biases presents a complex challenge.
Various measures can be taken to address bias in AI, such as diversifying and making datasets more representative during development, identifying biases early in this phase, adjusting algorithms post-development, or ensuring diverse composition of development teams. Additionally, particularly when the goal is to empower the general population, it is important to reflect on these developments. By increasing transparency and explainability in AI systems, users can better understand how decisions are made and identify potential biases. It is crucial to keep this in mind and critically evaluate information provided, consulting alternative sources if necessary. That something is already happening is demonstrated by the friendly prompt from ChatGPT: “If you notice any potential biases in my responses, please feel free to point them out, and I’ll do my best to address them.”
Read more
Joy, Buolamwini, & Gebru,Timnit, (2018). Gender Shades: Intersectional Disparities in Commercial Gender Classification. Proceedings if Machine Learning Research 81, 1-5. https://www.media.mit.edu/publications/gender-shades-intersectional-accuracy-disparities-in-commercial-gender-classification/
Sun, Tony, Andrew Gaut, Shirlyn Tang, Yuxin Huang, Mai ElSherief, Jieyu Zhao, Diba Mirza, Elizabeth Belding, Kai-Wei Chang, William Wang (2019). Mitigating Gender Bias in Natural Language Processing: Literature Review. https://www.researchgate.net/publication/333971854_Mitigating_Gender_Bias_in_Natural_Language_Processing_Literature_Review